Cutting through boundaries
Yuansi Chen joined the Department of Mathematics as an associate professor of statistics at the beginning of this year. His research interests are centred around problems in Bayesian statistics and machine learning, but cross disciplinary boundaries – a theme that is also reflected in his teaching.
Yuansi Chen is someone who loves to explore. At the age of 18, he moved from his native China to France, first to attend the Lycée Hoche in Versailles in preparation for the entry into the grandes écoles, and then to study at the École Polytechnique from 2010 to 2013. This was a time when machine learning (ML) and artificial intelligence (AI) were beginning to boom. Chen plunged into the mathematical intricacies that this fast-moving field presented and was intrigued – so much so that he decided to pursue the topic for his PhD, in the group of Bin Yu at the University of California, Berkeley (US).
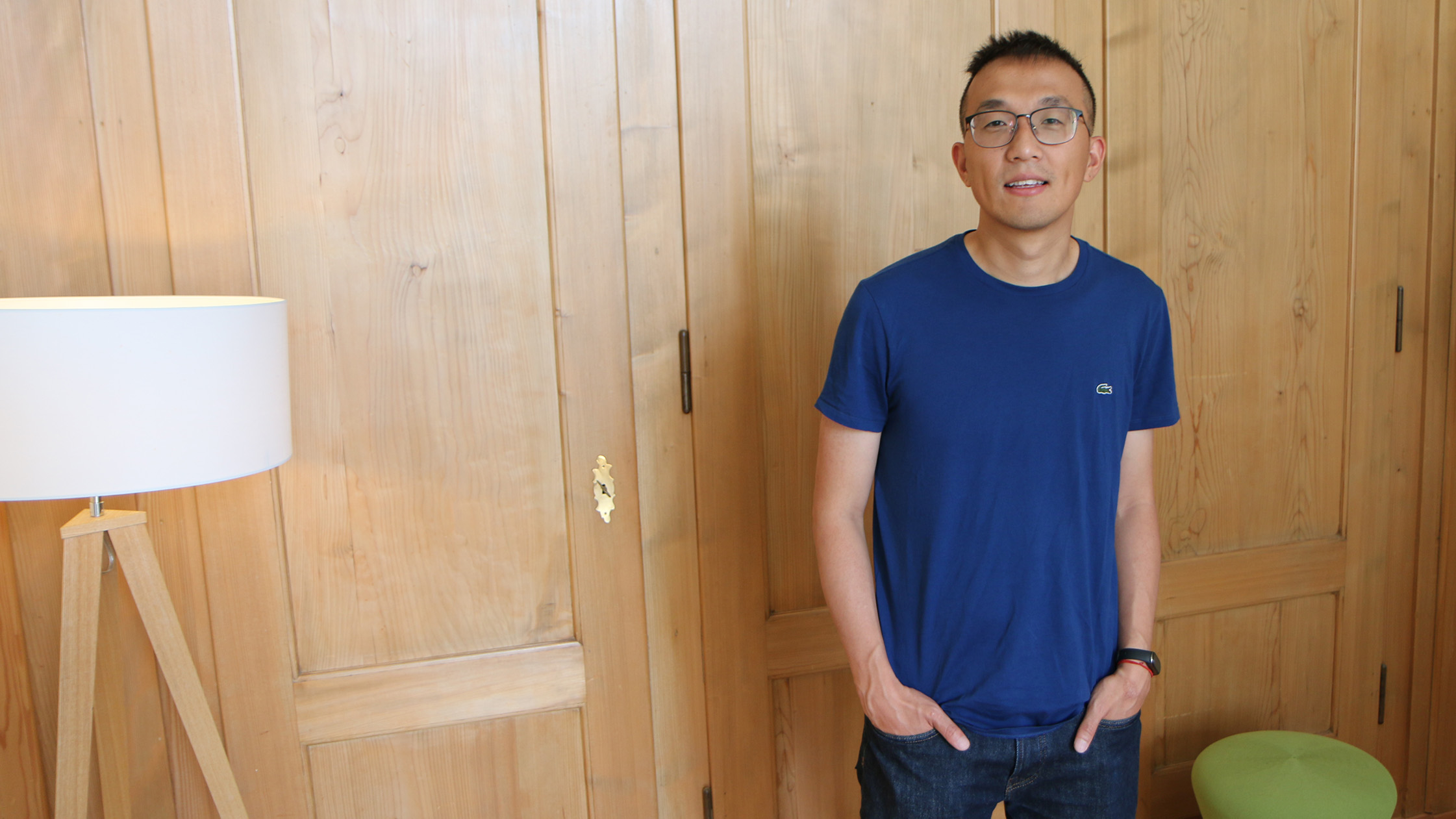
One of the questions he set out to tackle was how to efficiently generate random samples from a known distribution. This is a computational challenge not only in AI and ML, but also in operations research, statistics and a whole range of other areas involving stochastic models. Chen began to study so-called Markov Chain Monte Carlo (MCMC) algorithms, and gradually a hunch emerged about what might be limiting their performance. "We started to realize that the real bottleneck for such algorithms is the geometry of the problem," says Chen. That realization led to a breakthrough result that would attract widespread attention.
Research at the cutting edge
Exploring the connections between the computational complexity of sampling algorithms and geometry – convex geometry, to be precise – proved to be both challenging and fruitful, says Chen: "Convex geometry is a field quite distant from computational complexity, and when I got into the literature, it was difficult to read at first. But I ended up appreciating many intuitions and many creative ideas in this, for me, new field." In particular, this journey led him to the so-called Kannan–Lovász–Simonovits (KLS) conjecture.
The KLS conjecture is related to the question of how a convex shape is sliced into two equal parts using the shortest cut. Chen's creativity shines through when he explains the problem: "Imagine you have to cut pieces of cheese into equal halves while minimizing the exposed surface area, to keep the cheese as fresh as possible." For a disc-shaped Camembert, the solution is unsurprising: A straight cut through the centre of the wheel. For a wedge of cheese, however, the optimal cut is a less intuitive one: it is not a straight line, but a curved one (see the video). The real challenge now is to find the best cut, including curved ones, in higher dimensions. The problem is daunting and highly relevant at the same time; in particular, as smallest cuts in convex shapes are associated with bottlenecks in dynamical problems, limiting how fast, say, a random walker – or the smell of cheese – can travel.
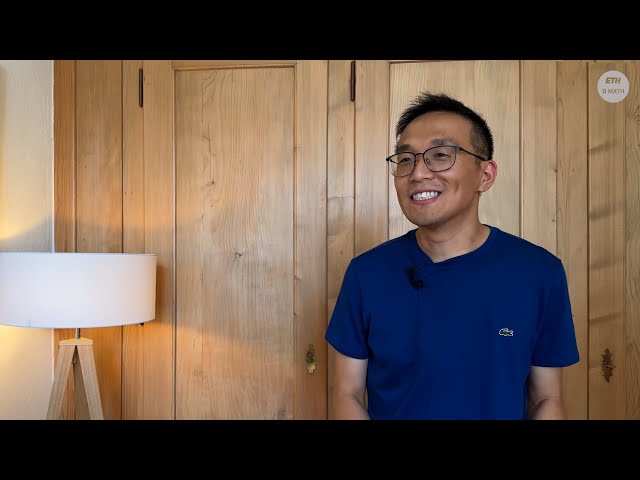
This extension to higher dimension is the problem that underlies the KLS conjecture, which was formulated in 1995, building on Jean Bourgain's 1984 'slicing problem' in convex geometry. The key question that kept researchers busy for decades was how fast the number of 'hidden' bottlenecks that arise from curved cuts (relative to straight-line cuts) grows as the dimension of a convex shape increases. Chen may have come to the problem as an outsider, but he was the one who succeeded in proving that there is an almost constant lower bound. In short, he showed that the complexity grows only very moderately with increasing dimension. This was an powerful and long-sought-after result in convex geometry, but it also had direct implications for a wide range of applications, for instance by providing statistical guarantees for how fast different algorithms can provide truly random sampling.
Chen's breakthrough result, published in 2021, received widespread attention and recognition, including invitations to speak in the Simons Institute Breakthroughs lecture series and the Nirenberg Lectures in Geometric Analysis in 2021. Chen had by then completed his PhD and came in July 2019 as a postdoctoral researcher to ETH Zurich, where he worked in the Seminar for Statistics and at the ETH Foundations of Data Science, under the supervision of Peter Bühlmann. In March 2021, he took up the position of an assistant professor at Duke University (US), where he received several prestigious grants and awards, among them a Sloan Research Fellowship, an NSF CAREER Award and a Ralph E. Powe Junior Faculty Enhancement Award, before returning to Switzerland in January 2024.
“There might be some kind of culture shock at the beginning, but eventually you come to appreciate the new culture.”Yuansi Chen about venturing into different areas of mathematics
Cherished 'culture shocks'
Chen compares living in different countries to venturing into different areas of mathematics: "There might be some kind of culture shock at the beginning, but eventually you come to appreciate the new culture." As an example he turns again to the subject of cheese: "I had never eaten cheese before coming to Europe. The first time I tasted Camembert poêlé in Paris was a shock: both the pungent smell and the rich and indulgent taste. Later, I fell in love with different types of cheese; beyond the pleasure of eating delicious food, it is a way of getting to know about the people, the geography and the climate of the regions where the products come from."
In the context of his research, Chen is keen to take the younger generation with him on the journey across disciplines (whereas in the cultural explorations he is joined by his wife and their one-year-old daughter). In the past spring semester, he introduced the Master and PhD-level course "Diffusion Models, Sampling and Stochastic Localization", which prepares students for academic research in current topics at the intersection of statistics and computer science, such as MCMC sampling algorithms and diffusion generative models. The course has attracted considerable interest from students in D-MATH and D-INFK alike. And given the many further connections Chen sees between applied statistics and various other fields, his quest for new research ideas – and possibly new course offerings – is unlikely to end any time soon.